What are Rosenshine's Principles of Instruction?
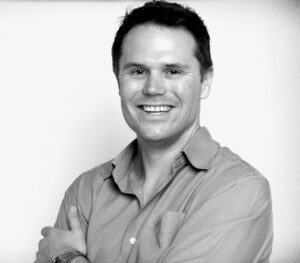
Bill Hansberry
Co-Director Playberry Laser
Barak Rosenshine, an American educational psychologist, developed Rosenshine’s Principles of Instruction. His principles are based on empirical research and observations of effective teaching practices. These principles aim to provide teachers with a framework for effective instructional strategies. Rosenshine began work in the 1970s and 1980s, conducting studies and synthesising research findings on effective teaching methods. His work culminated in identifying principles that he observed to be present in successful classrooms.
Rosenshine’s Principles of Instruction have gained momentum, prompting us all to consider whether our teaching is compatible with human cognitive architecture. Their common sense and simplicity strike anyone who reads them. Rosenshine’s Principles provide a clear roadmap for improving students’ retention and application of what we teach them.
Rosenshine’s Principles of Instruction reflect fundamental elements that have stood the test of time and are rooted in the foundations of successful teaching practices. For me, Rosenshine’s principles are highly congruent with the methodologies for teaching individuals with dyslexia developed by Samuel Orton and Anna Gillingham in the 1920s. The enduring nature of these effective teaching principles underscores that regardless of educational advancements and evolving methodologies, certain fundamental elements of excellent instruction remain constant.
Over this series of posts, I will lay out each of Roshenshine’s ten principles as outlined in an article Rosenshine wrote for American Educator in the Spring of 2012 and elaborate slightly on each of these, bringing some of my thoughts and insights to them, particularly in how they relate to how many of us in the evidence-informed teaching of literacy space have relearned to teach structured literacy lessons and how this explicit and routine heavy teaching has successfully spilt into other curriculum areas. I want to show how we’ve been adhering to these principles of instruction all along.
Principle 10: Engage students in weekly and monthly review: Students need to be involved in extensive practice in order to develop well-connected and automatic knowledge.
“Research Findings
Students need extensive and broad reading, and extensive practice in order to develop well-connected networks of ideas (schemas) in their long term memory. When one’s knowledge on a particular topic is large and well-connected, it is easier to learn new information and prior knowledge is more readily available for use. The more one rehearses and reviews information, the stronger these interconnections become. It is also easier to solve problems when one has a rich, well-connected body of knowledge and strong ties among the connections… (Rosenshine)
I write about this tenth and final principle, feeling a bit like a kid in a candy shop. I’m just coming down from multiple listens to Greg Ashman talking with John Sweller about Cognitive Load Theory on his Filling the Pail podcast, and just when I thought I’d had my fix, a colleague alerted me to Greg Ashman (again) talking on the Science of Reading Podcast about (you guessed it) Cognitive Load Theory. Dyscastia episode 15 had David Morkunas talking about retrieval practice. Michael and I just interviewed Churchill Fellow – Liana McCurry for Dyscastia episode 17 (coming soon) on what she saw high-performing countries doing in the maths teaching space. And we talked a lot about (you guessed again) Cognitive Load Theory, retrieval practice, automaticity and fluency with number facts.
Seriously, I cannot get enough of this stuff. I’ve become a human cognitive architecture, cognitive load, retrieval and automaticity junkie. I need help. Is there a group for people like me?
So, here we go. I’ll try to keep this briefer than the last one, but no promises! I want to start with knowledge and the place it’s kept – long-term memory. Greg Ashman said something in the Science of Reading Podcast that was immediately written in Sharpie on the inside of my windshield.
“Knowledge is what you think WITH.”
The inside of my windshield is where all the phrases I want to remember go! So, congratulations, Greg Ashman. You’ve made it onto my windshield with other greats like Dan Willingham and Anita Archer (although they’ve since faded a little, they’re alive and well now in my long-term memory and getting lots of retrieval practice).
To put this in context, this was part of a broader statement:
‘This idea that kids don’t need to know anything anymore; they just have to practice skills is really quite a pernicious and damaging idea. They do need lots of knowledge in long-term memory if they are going to be able to think in a sophisticated way, and develop critical thinking skills because…Knowledge is what you think WITH.
These five words powerfully sum up why high-performing countries use knowledge-based curricula (instead of skills-based curricula). These five words explain why the notion that we shouldn’t teach kids knowledge is garbage AND why inquiry-based teaching is utterly useless until students have a robust knowledge base (lots of chunked, automated and integrated bits of knowledge and procedure) to enquire from. It also demolishes the idea that we should try to teach children to think like experts – inquire like scientists or mathematicians, write like authors … blah blah blah!
I talked about the differences between the long-term memories of novices and experts in Principle 4. You may remember the example of the Chess masters and why they routinely beat less experienced players – more chess stuff in their long-term memory. Experts have larger and better-connected patterns of chess knowledge (knowledge networks/schemas) that actually free up their working memory so they have mental workspace to bring more to their game – notice their opponent’s body language, perhaps better anticipate their next move. The expert’s working memory is easily accessing a network of stored board configurations, and no matter how the board looks, because there are 10,000+ hours of experience stored as vast knowledge networks in their long-term memory, the expert won’t see much on a chess board that won’t trigger that “I’ve seen this before, and I know where to next” feeling.
They use this knowledge to think about their next move.
You simply can’t take a novice chess player and teach them to think like a master. They don’t have the equipment. Their long-term memory for chess looks nothing like the experts. There’s just no hack or shortcut to that. Similarly, you can’t take a school student and teach them to problem solve like a mathematician. They don’t have the equipment in long-term memory.
An Expert mathematician, when working on something not yet discovered or solved, is able to hold in mind and process the really hard stuff involved with the problem (mentally move it around in their working memory) and, while doing this, have NONE of that precious working memory space taken up by recalling multiplication facts, factors, prime and composite numbers, or index laws that are needed to do the problem-solving. Those lower-order skills simply appear in working memory effortlessly and get thrown into thinking mixing bowl with the hard stuff at no cost to working memory!
The less experienced (or novice) mathematician, when faced with the same problem, will have to use up valuable space in their working memory to calculate a set of factors, think about whether a number is prime or not or maybe quickly google index laws, thereby, bringing new information from the environment into working memory to be processed. All of this lack of automaticity with the stuff the expert could retrieve and use effortlessly overloads the novice’s working memory, and they lose the picture of the bigger problem they’re trying to solve. This, my friends, is called cognitive overload.
Let me give you a sport-skill example. I know it’s a little inexact and an expert on human movement may see some inaccuracies in how I have represented motor working memory, but for our purposes, it works.
The expert tennis player Novak Djokovic effortlessly retrieves from long-term all the millimetre-perfect movements needed for a topspin cross-court backhand.
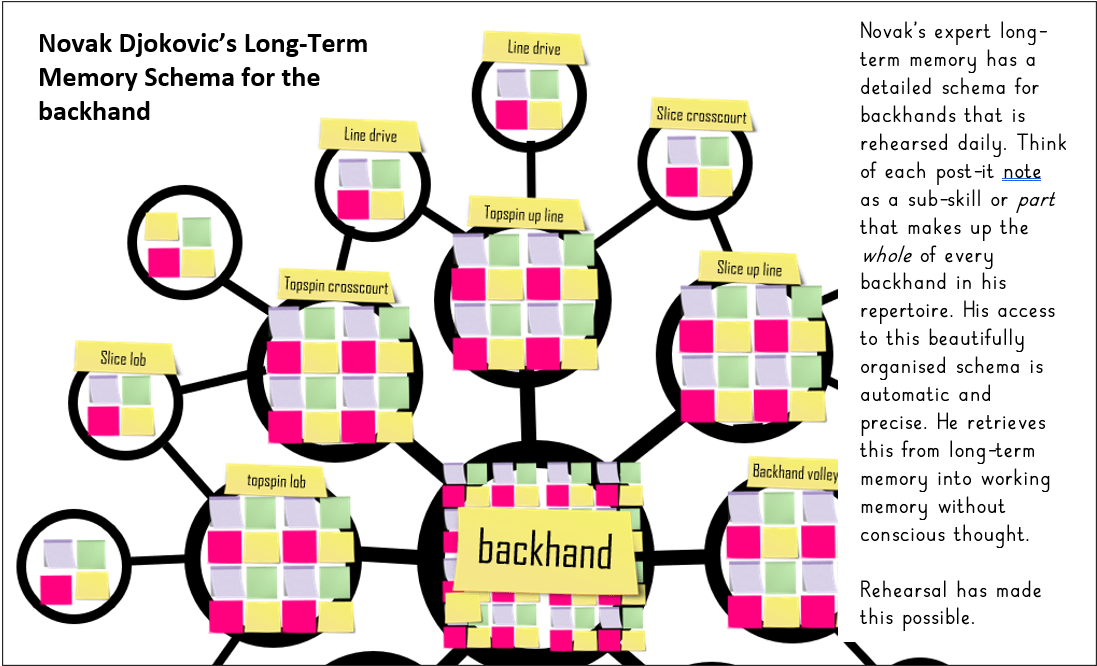
The stroke is performed over and over as a seamless motor routine. The ball rarely hits the net or goes out. Novak’s crosscourt topspin backhand can be thought of as an automated chunk. He doesn’t have to consciously think about this stroke as he plays it (which would use working memory) – it just happens near-perfectly and automatically.
What does this automaticity mean for how Novak’s working memory is used during a point? It means that he can play this stroke, and at the same time, he can utilise his working memory to watch and think about what his opponent is doing.
Imagine that as the ball comes toward Novak he begins to position his body and feet and starts his backswing to play a crosscourt backhand. Just as Novak starts his backswing, his opponent slips a little. Because preparing for the backhand is automated for Novak (no conscious thinking required), he has some working memory available to process this. He quickly changes his shot choice and drives a backhand up the line. Winner! Novak had mental space left over to process new information and use it to his advantage.
Put me in the same position, and my working memory would be at capacity as I mentally recite to myself and consciously think about the basics of getting a topspin backhand over the net and into the court:
“Watch the ball, racket back early, push onto the front foot, hit up the back of the ball!”
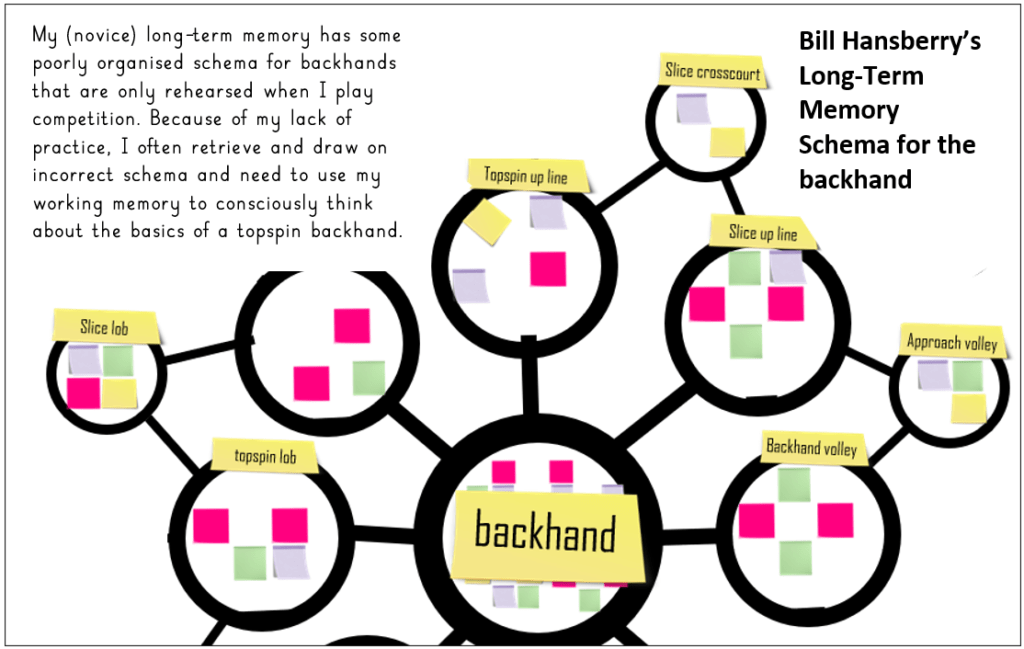
My opponent could fall flat on their face, and I wouldn’t know. I’m a novice; Novak is an expert. He thinks very differently to me on a tennis court, and I have no shortcuts to that level of expertise.
How did Novak get to this point of expertise?
‘The best way to become an expert is through practice-thousands of hours of practice. The more practice, the better the performance.’
This diagram by Oliver Caviglioli is a beautiful summary of human cognitive architecture. It shows the relationship between the environment, attention, working, and long-term memory. The tree-like structure represents long-term memory. Look above at Novak’s and my long-term memory (LTM) for the backhand, and you see how I’ve used this diagram.
The lines show information entering long-term memory (LTM) from working memory (WM) (learning) and being pulled from LTM to be used in WM (remembering or retrieval). Take a moment to have a good look at this model, as I will use it to discuss review and practice.
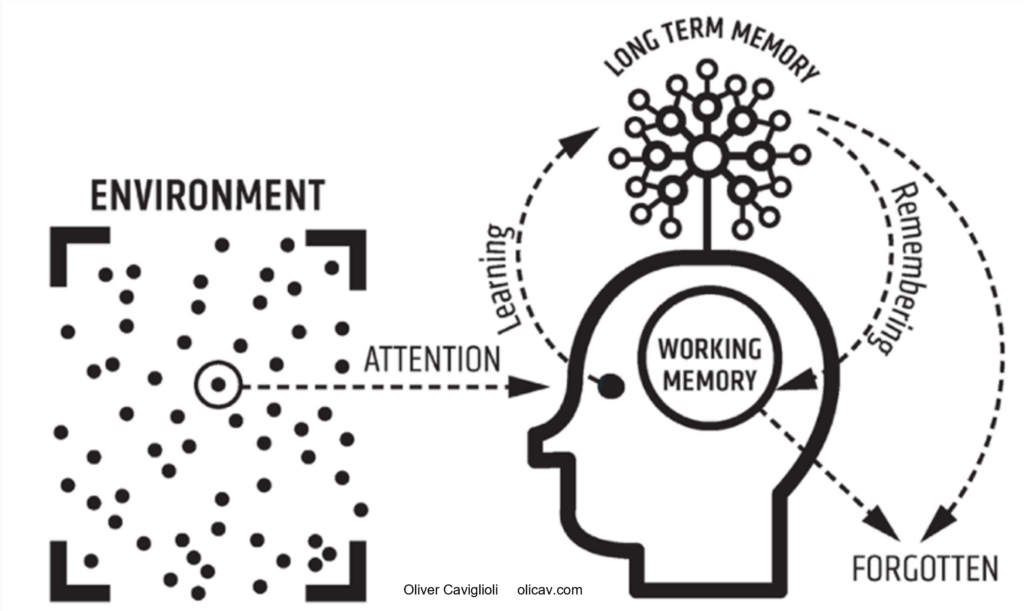
This model represents a number of important parts of the cognitive (thinking) system and, most importantly, how information (knowledge) we use to think with comes into working memory to be processed and used from either the environment or our own long-term memory. When Novak played the winning backhand one important piece of information from his environment was his opponent slipping. Novak’s LTM had an incredibly dense and well-organised bank of tennis knowledge, including schema for the perfect backhand that he used to think about what to do when his opponent slipped.
“Knowledge is what you think WITH.”
Working Memory
Conscious thinking takes place in working memory, which is a very small workspace. I alluded to this in the examples above—the chess expert, the mathematician, and the tennis expert Novak Djokovic and their less-expert (novice) equivalents.
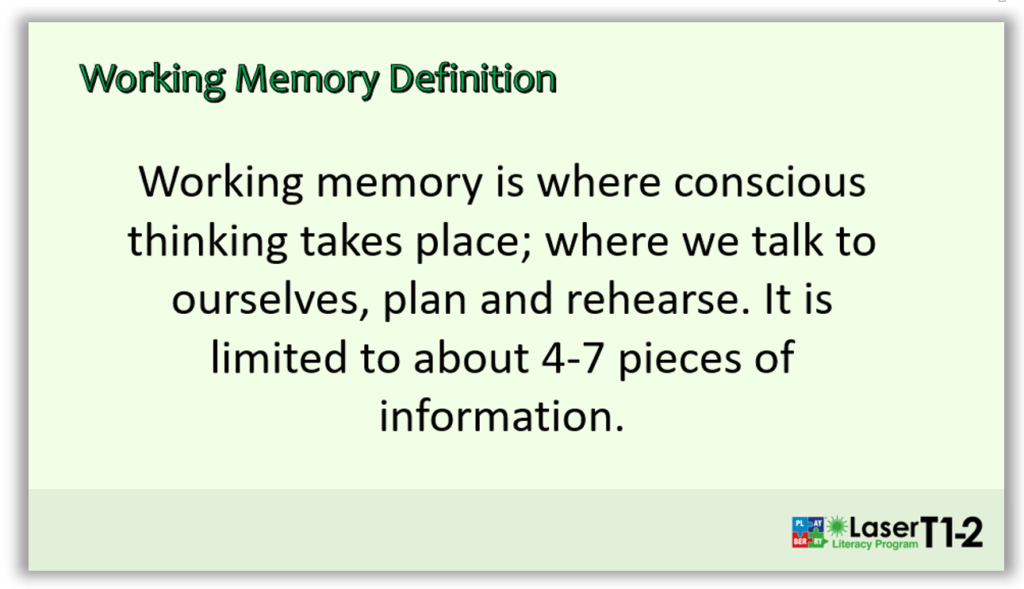
In each example, there was no difference between the experts and the novices in terms of working memory capacity. No matter who you are, no matter how good you are at something, you, me, the chess master, the mathematician and Novak have a working memory that is limited to being able to deal with (process) around 4 items of new (novel) information at a time. For some, it’s a couple of items less; for others, it’s a couple more. The important point is that we’re all dealing with an incredibly limited mental workspace to think, talk to ourselves, plan and mentally rehearse.
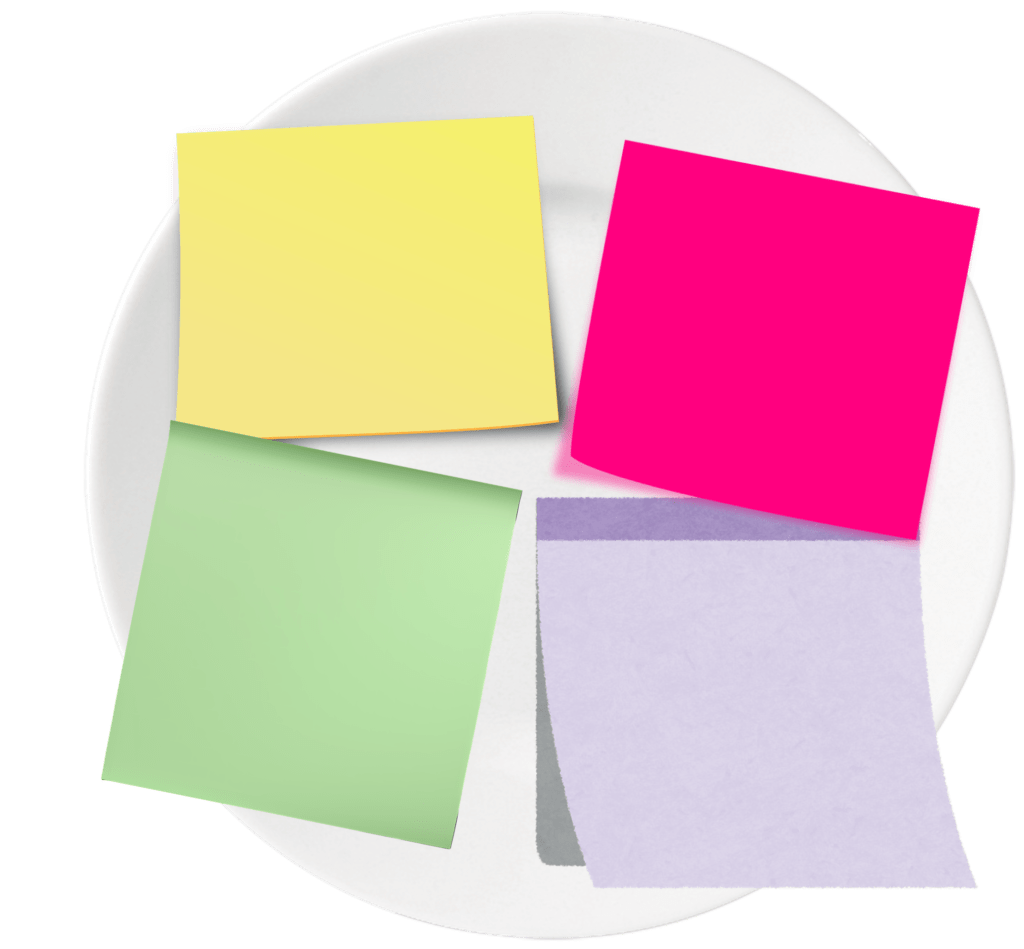
If you think of your working memory being about the size of a bread plate and each item of novel information as the size of a Post-it note, That’s not much space!
If you try to introduce another Post-it to the plate, one will fall off. That’s why you forget simple things when you have a lot on your plate—that’s cognitive overload. Pun is absolutely intended!
Some experts refer to working memory as a bottleneck in thinking, and this is a pretty good analogy. New information comes into working memory from the environment; however, because the plate is small, only a few items of information (about 4) can be processed at one time.
Once they are processed, they make their way into long-term memory and become integrated into vast knowledge networks (schemas). In my diagram, items of information are represented as post-its. Think of each Post it as one piece if information. This information can be little pieces of how to do something (procedural knowledge) or information in the form of facts (declarative knowledge). When Novak quickly changed his stroke choice as the ball flew at him and his opponent slipped, he drew on already stored procedural and declarative knowledge, pulled it into working memory to be thought with and hit a winning backhand up the line.
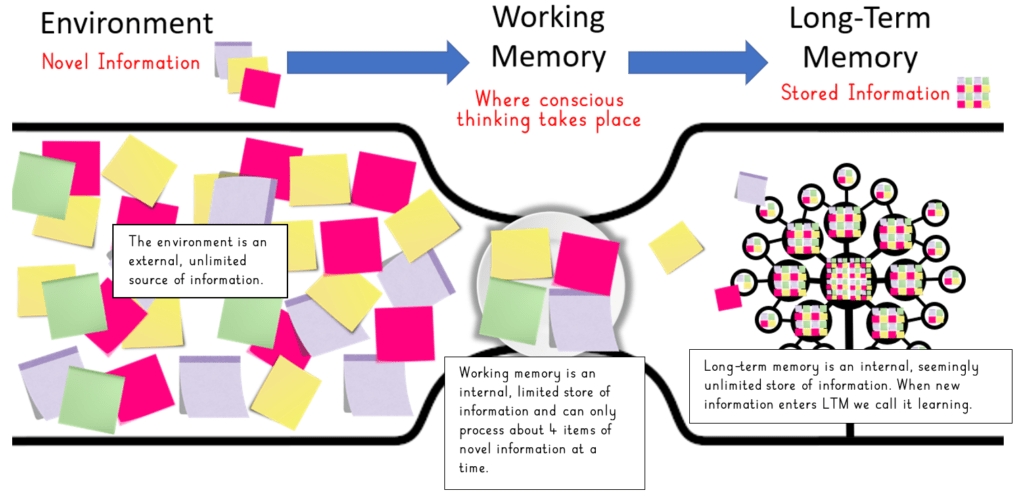
So, what accounts for the huge difference in performance between experts and novices on similar tasks? Experts have more room in their working memory to do complex tasks and think complex thoughts. Novices do not. Remember Novak and the backhand? He made a last-second decision to play that shot because he had working memory space available to notice his opponent’s small slip and act on it. The information needed for the backhand, with all of its intricate movements, came into working memory and was executed perfectly.
Novak didn’t have to consciously think: Watch the ball, racket back early, push onto the front foot, hit up the back of the ball like I do when I want to perform the same shot. Why? Because Novak has practised that shot and its sub-components tens of thousands of times. The small pieces of the backhand skill are chunked together in Novak’s long-term memory as a seamless and complete routine. This routine has also connected itself to other chunked and automated routines that contain some sub-components that are the same (like a cross-court backhand).
Novak has a well-used network in long-term memory of all sorts of different backhands for different situations. Because these schemas are accessed so often, retrieving information from them is fast and reliable.
The sub-routines of Watch the ball, racket back early, push onto the front foot, hit up the back of the ball are chunked and automated into a larger routine, and when the moment is right, when Novak recognises a particular moment– a particular configuration of him and his opponent on the court that lends itself to a backhand up the line, Novak draws from long-term memory an automated routine for that particular backhand. That routine enters working memory but takes up almost no space. Why?
Because knowledge stored in long-term memory takes up almost no space when it moves back into working memory to be processed along with novel information from the environment.
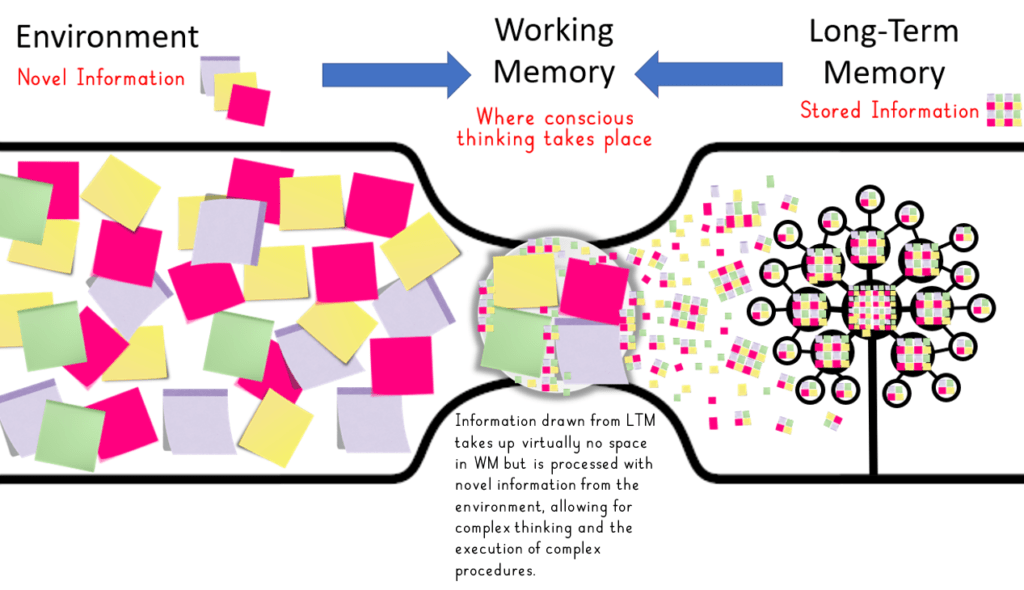
Information from long-term memory does not occupy one of those 4 available places on the plate. Novak’s plate was clear enough for him to think about other information coming from the environment as he prepared to play the shot and execute it.
Every time Novak activates one of these schemas in a slightly different (novel) situation, a new network (skill) begins to form, which, if used (rehearsed) enough, integrates into Novak’s backhand schema.
“Knowledge is what you think WITH.”
Rosenshine’s 10th reminds us of the importance of broad reading and extensive practice in order to develop well-connected networks of ideas (schemas) in long-term memory (LTM). My chess master, mathematician and tennis pro examples above explain how well-developed schemas (lots of knowledge) in long-term memory allow humans to overcome the limits of their working memories when they are faced with new situations and problems to be solved. Practice as well as weekly and monthly review of previously learned material makes for efficient and reliable retrieval.
Review and Retrieval of information from Long Term Memory
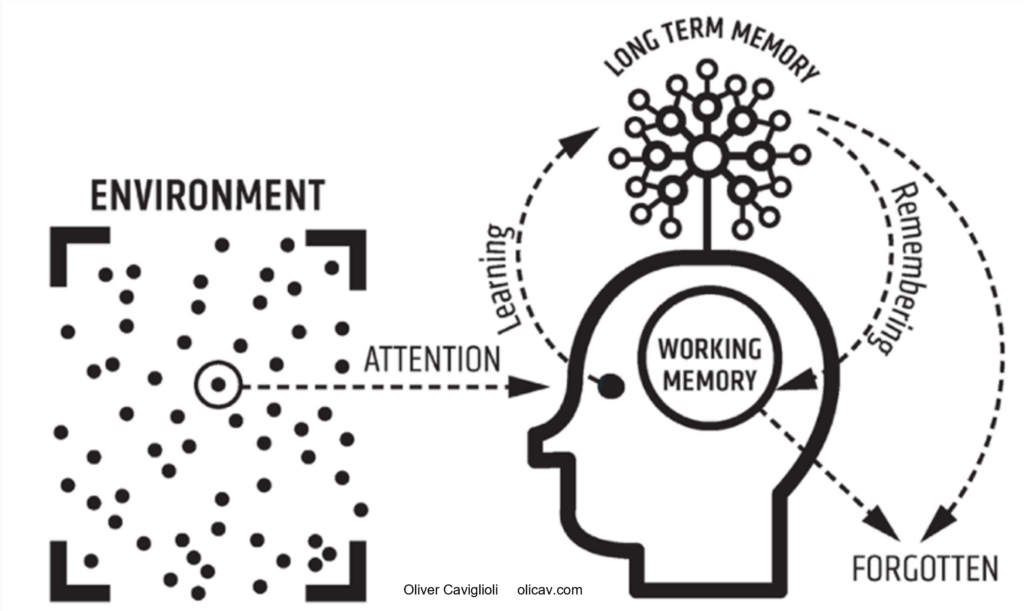
Weekly and monthly review makes it easier for our brains to retrieve (remember) information that has been stored in long term memory. When we learn something new that we have little background knowledge of, our initial practice (retrieval) attempts of that new learning are difficult and error-prone. Retrieving and using the newly learned information will feel like finding our way across a grassy field in thick fog.
Retrieval is like walking the same way over and over. Eventually, our faint, barely visible footmarks become light spots of flattened grass. With even more trips along that path (retrievals), we’ll form an unmistakable, well-worn path where the grass no longer grows. This is analogous to how the brain creates neural pathways for retrieving information from long-term memory; the well-worn pathways (the ones that get used often) become stronger. Because the brain operates on a use it or lose it principle, the less retrieved information paths become overgrown again, like we’ve never been there – the neural path is abandoned and disconnected by the brain. The information has been forgotten.
Rosenshine also makes the point (that I didn’t go into) that when one’s knowledge on a particular topic is large and well-connected, it is easier to learn new information, and prior knowledge (stored in LTM) is more readily available for use. He states that the more one rehearses and reviews information, the stronger these interconnections in LTM become, making it easier to solve problems.
A Word on Curriculum
Currently, there is debate about different types of curriculum – skills-based vs knowledge-based. Skills-based curricula focus on teaching students thinking skills and learner dispositions that are believed to be applicable across knowledge areas (domains). This way of thinking is underpinned by a belief that teaching students facts, figures and procedures (knowledge) is a waste of time when students can just google knowledge. Educational futurists trot out narratives about how human knowledge grows at such a rate that there’s no point teaching knowledge and it’s better to teach thinking skills and dispositions. Sounds intuitive, but it turns out that the poorest performing countries in educational outcomes (Australia included) have a strong bias toward this view of learning and skills-based curricula. The Education Research Reading Room podcast has an interesting episode with curriculum expert Ben Jensen that discusses skills-based vs knowledge-based curriculum.
Skills-based curricula are politically safe because they let authorities off the hook for making decisions about what knowledge schools should teach. These issues are highly politicised and often polarising. Consider the debate about how Australia’s colonisation should be taught or debates in the US about whether states should teach students a broader notion of gender.
The opposite of a skills-based curriculum, a knowledge-based curriculum subscribes to the idea that knowledge is hugely important. It posits that building deep and well-connected schemas in students’ long-term memories on a range of domain-specific areas sets students up to be successful learners, critical thinkers, and good problem solvers because knowledge is what you think with. This type of curriculum demands that educators be experts in what they teach and also demands very detailed curriculum documentation and more direct and explicit methods of teaching this knowledge.
There’s no surprise which type of curriculum Rosenshine’s research would support.
Playberry Laser T1-2 is a teacher-supportive multisensory literacy resource for primary teachers to support their teaching in line with research. We’ve taken the planning and resource design load to free teachers to focus on building content knowledge and sharpening their delivery in line with Rosenshine’s Principles of Instruction.
References:
Rosenshine, B. (2012). Principles of instruction: Research-based strategies that all teachers should know. [online] American Educator, American Educator, pp.12–39. Available at: https://www.aft.org/sites/default/files/periodicals/Rosenshine.pdf
Sherrington , T. (2019). Rosenshine’s Principles in Action. John Catt
3 Responses
If you find a support group for junkies of human cognitive architecture, cognitive load, retrieval and automaticity can you please let me know. I’ll need to join too! 😉
Love you work Bill.
Ash
Shall we start the group?
Shall we start the group?